GIS 4036 - Unsupervised and Supervised Image Classification
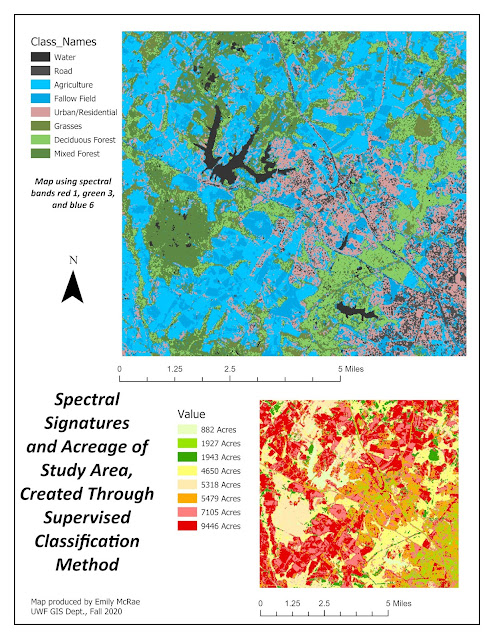
This module focuses on methods of classifying raster images, including supervised and unsupervised classifications. The above map shows an example of supervised image classification completed in ERDAS IMAGE and mapped in ArcGIS Pro. By creating features in an image to feed an algorithm, an image was classified based on several polygons created manually. These images were matched across the entire image to create classes. Spectral bands were then compared through histograms to identify the bands which need to be separated due to overlap in like features. The image was then displayed based on these classes, as well as acres belonging to that class.